[作者-王欣芮|审核-刘心月]近日,人工智能领域顶级国际会议ICLR-2025(The Thirteenth International Conference on Learning Representations)公布论文接收结果,本组的两项研究成果被录用。
论文:Towards Generalization Bounds of GCNs forAdversariallyRobust Node Classification
论文概述:图卷积神经网络的对抗鲁棒泛化性已经受到安全至关重要领域的广泛关注。尽管取得了极大经验性进展,图卷积神经网络面临对抗攻击时的泛化行为的理论理解仍然鲜有研究。为了揭露这一谜题,本文利用转导Rademacher复杂度和新颖的图卷积收缩技术在节点分类的背景下建立了图卷积神经网络的高概率泛化界。给出的界限捕捉了泛化误差与对抗扰动直接的作用关系,揭露了一些缓解扰动负面影响的重要因素,例如低维的特征投影,扰动依赖的范数正则化器,归一化图矩阵以及合适的网络层等。此外,本文提供了适用于广泛图卷积模型在r≥1加性扰动攻击性的基于TRC的误差界。理论结果的比较证实了特定网络架构能够有助于缓解扰动前向传播的累计效应。基准数据集上的实验结果证实了本文的理论发现。
【英文摘要】Adversariallyrobust generalization of Graph Convolutional Networks (GCNs) has garnered significant attention in various security-sensitive application areas, driven by intrinsic adversarial vulnerability. Albeit remarkable empirical advancement, theoretical understanding of the generalization behavior of GCNs subjected to adversarial attacks remains elusive. To make progress on the mystery, we establish unified high-probability generalization bounds for GCNs in the context of node classification, by leveraging adversarialTransductiveRademacher Complexity (TRC) and developing a novel contraction technique on graph convolution. Our bounds capture the interaction between generalization error and adversarial perturbations, revealing the importance of key quantities in mitigating the negative effects of perturbations, such as low-dimensional feature projection, perturbation-dependent norm regularization, normalized graph matrix, proper number of network layers, etc. Furthermore, we provide TRC-based bounds of popular GCNs with lr-norm-additive perturbations for arbitrary r≥1. A comparison of theoretical results demonstrates that specific network architectures (e.g., residual connection) can help alleviate the cumulative effect of perturbations during the forward propagation of deep GCNs. Experimental results on benchmark datasets validate our theoretical findings.
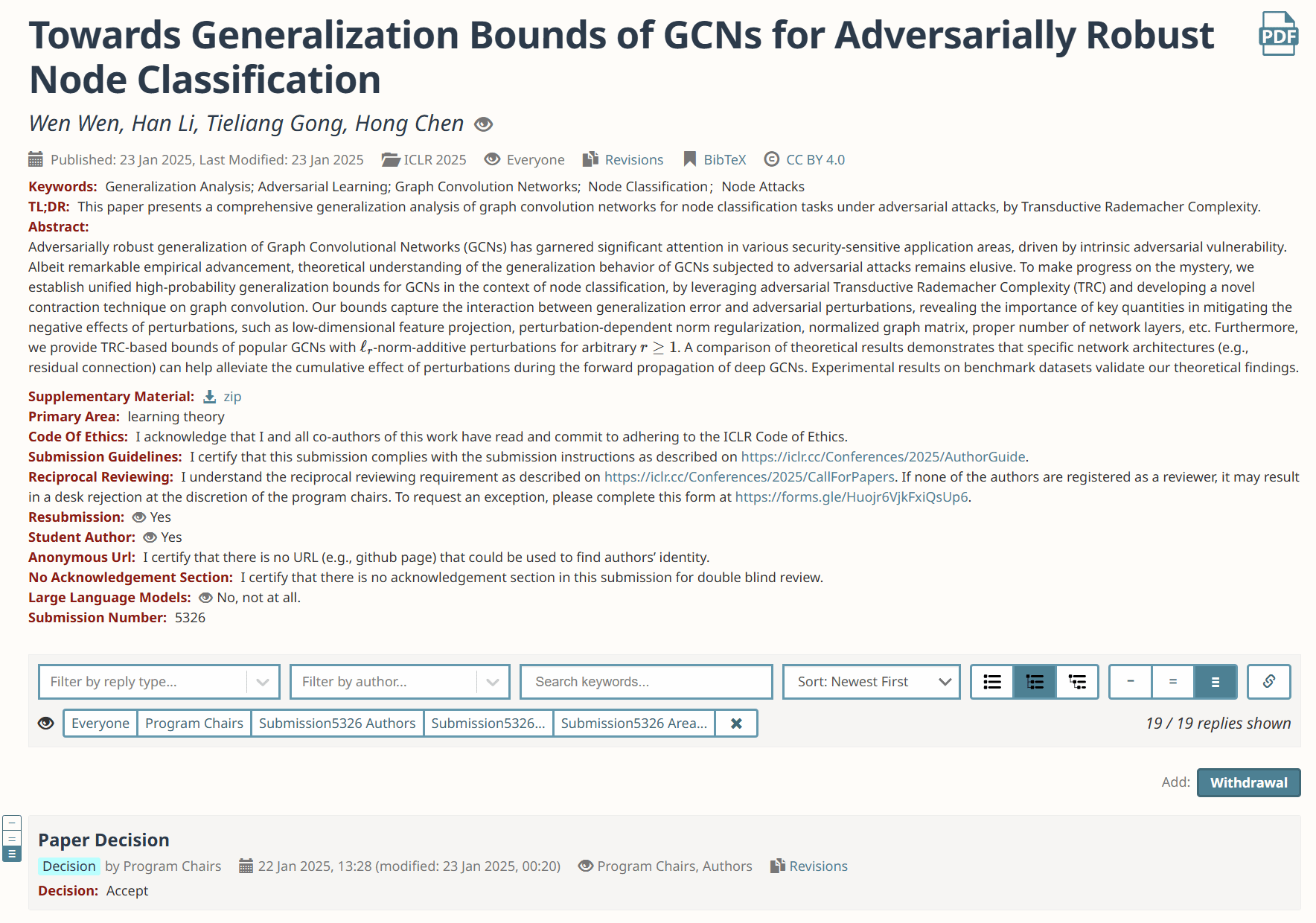
论文:A Statistical Approach for Controlled Training Data Detectio
论文概述:检测大型语言模型(LLM)的训练数据正受到越来越多的关注,特别是在需要高可靠性的应用中。虽然已经做出了许多努力来解决这个问题,但它们通常只关注准确性,而不确保可控的结果。为了填补这一空白,我们提出了一种基于敲除推理的训练数据检测器(KTD),这是一种在训练数据检测中实现严格错误发现率(FDR)控制的新方法。具体来说,KTD生成合成的仿冒样本,无缝替换原始数据点,而不会损害上下文的完整性。然后计算一种新的敲除统计量,该统计量包含多个敲除抽取,以确保FDR控制,同时保持高功率。我们的理论分析证明了KTD在FDR控制和功率方面的渐近最优性。在WikiMIA、XSum和实时BBC新闻等真实世界数据集上的实证实验进一步验证了KTD与现有方法相比的优越性能。
【英文摘要】Detecting training data for large language models (LLMs) is receiving growing attention, especially in applications requiring high reliability. While numerous efforts have been made to address this issue, they typically focus on accuracy without ensuring controllable results. To fill this gap, we propose Knockoff Inference-based Training data Detector (KTD), a novel method that achieves rigorous false discovery rate (FDR) control in training data detection. Specifically, KTD generates synthetic knockoff samples that seamlessly replace original data points without compromising contextual integrity. A novel knockoff statistic, which incorporates multiple knockoff draws, is then calculated to ensure FDR control while maintaining high power. Our theoretical analysis demonstrates KTD's asymptotic optimality in terms of FDR control and power. Empirical experiments on real-world datasets such as WikiMIA, XSum and Real Time BBC News further validate KTD's superior performance compared to existing methods.
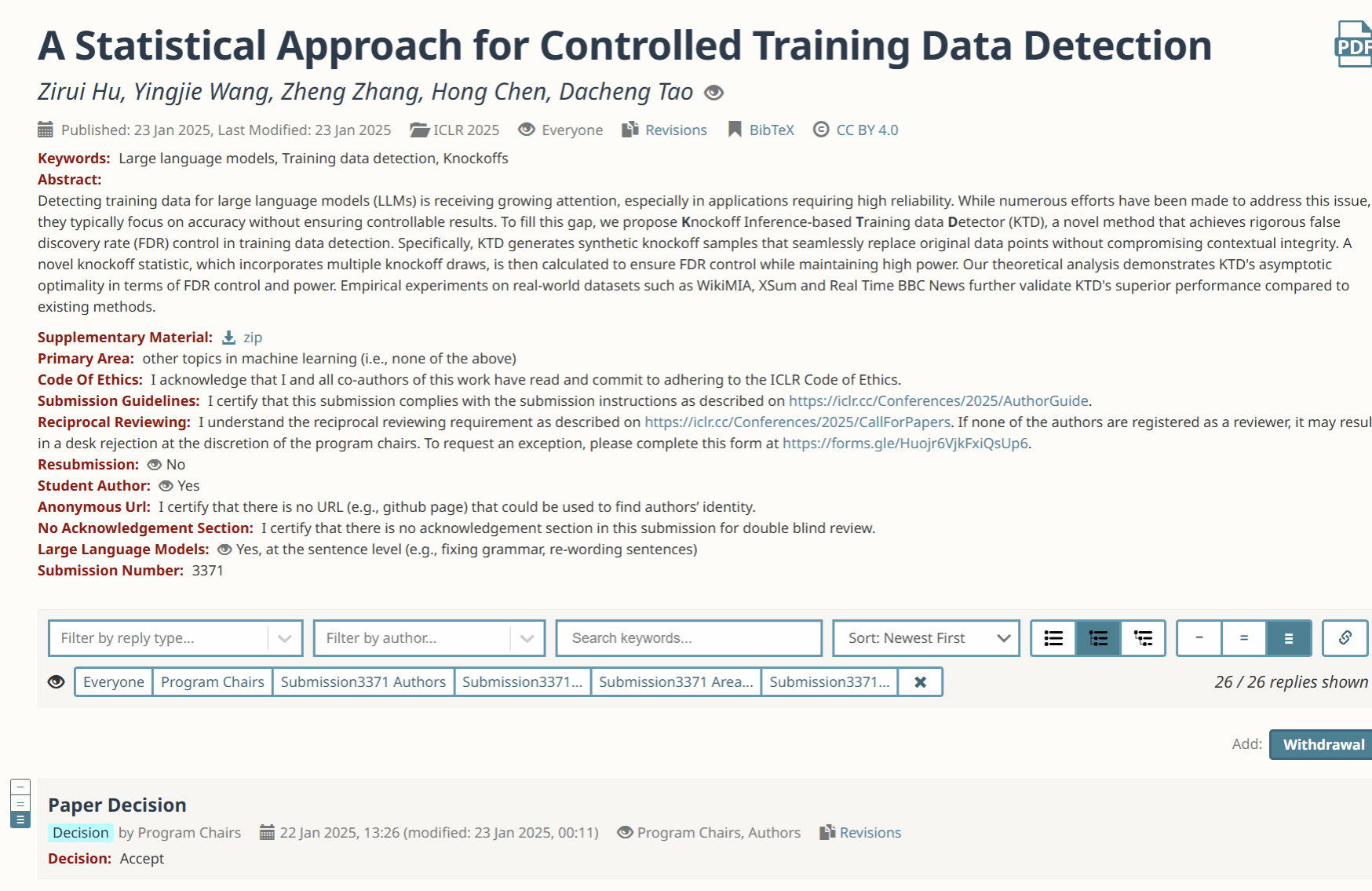
此次两篇论文的成功录用,离不开团队成员的辛勤付出和导师们的悉心指导。我们深知,这只是研究道路上的一个小进步,未来仍需不断努力。